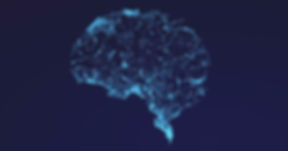
The Problem
The physiological conditions underlying psychiatric disorders are not well understood at present. Current psychiatric diagnoses rely on clinical observations and symptomatic criteria, leading to a lack of clear boundaries between different disorders, as well as high heterogeneity within the same disorder category.
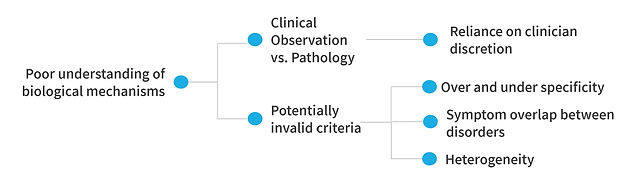
Our Goal
In the future, the hope is to have more robust diagnostic criteria, potentially based upon neuroscience and other biomarkers instead of behavioral characteristics. Eventually, this may lead to the development of additional diagnostic tests using a patient’s biological data.
Before that can be done however, the connection between biological systems and expressed symptoms must be established.
​
Our research question: do neuronal biomarkers correspond to current classifications of mental illness?
Project Approach

-
Develop Supervised and Unsupervised models
-
Use the models to visualize the data using clustering
-
Use the models to identify important areas of the brain